Connected Risks in a Connected World
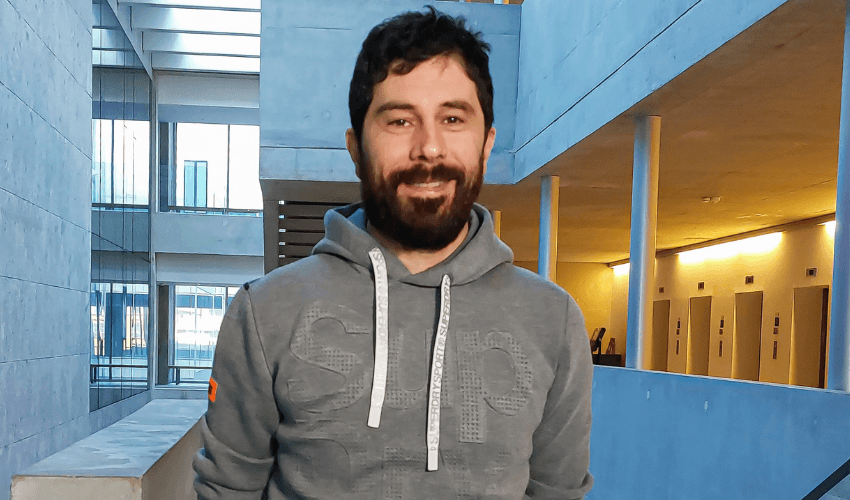
The speed at which COVID-19 has spread all over the globe has once again exposed how connected our world is: an event taking place in a single location is able to affect the rest of the planet in a short time. More and more often, these events are extreme, like exceptionally heavy rainfalls or unprecedented heat waves. Also, financial markets undergo similar shocks, with abrupt raises and falls in the value of stocks and currencies. In an increasingly connected world, these extreme phenomena are inevitably connected too. Hence, a joint analysis of extreme events, both natural and financial, is now more needed than ever.
In a recent paper, Simone Padoan, Professor at the Bocconi Department of Decision Sciences, and Gilles Stupfler (ENSAI Rennes, CREST) have proposed novel tools in extreme value theory to study multivariate extremes and have used these new methods to jointly analyze the exchange rates of the British Pound versus the United States Dollar, the Japanese Yen, the Canadian Dollar, the Australian Dollar, and the Norwegian Krone, over the last 40 years. Analyzing multiple exchange rates simultaneously can be useful in understanding and predicting the risks for nations and companies exposed to the global economy.
One of the most popular risk measures in finance is the so-called value at risk, which can be seen as a threshold that, when exceeded, should raise our concerns. Luckily enough, such a threshold is trespassed with a low probability; in other words, extreme events are rare. In probabilistic terms, the value at risk is essentially a percentile or, similarly, a quantile. A percentile is a threshold under which you can find a given percentage of the data; for example, 70% of the data in a dataset is smaller than their 70th percentile. The definition of quantile is similar, just allowing for different splits of the data. Even if the concept of quantile/percentile is simple and effective, it is not perfect. In particular, it generally lacks an important property in order to be a coherent risk measure, that is sub-additivity. In brief, sub-additivity requires that the risk of the sum of two quantities (for example, two stock prices in a portfolio) is smaller or equal to the sum of their individual risks. In the portfolio example, this corresponds to the diversification principle, according to which the risk of the whole portfolio cannot be higher than the sum of the risks of each individual stock position.
“This is why,” explains Professor Padoan, “we resorted to expectiles. While quantiles and percentiles generalize the concept of median (the value splitting an ordered sample in two equally-sized halves), expectiles generalize the arithmetic mean, arguably an even more familiar concept. More specifically, while quantiles and percentiles only rely on the ordering of the data, expectiles also account for their magnitude (technically, the expected distance among the data). Notably, despite being the extension of a simple concept like the arithmetic mean, expectiles allow us to tackle very complex research questions, such as the study of multivariate extremes, which is a hot topic in extreme value theory.”
“In fact, despite having a long history and a rich literature, extreme value theory has mainly focused on univariate extremes. However, our increasingly-connected world definitely needs to tackle the challenge of multivariate extremes, like the ones that we studied in this paper. The methodology that we developed can be employed not only in financial studies, but also for the much-needed assessment of environmental risks. In this sense, extreme value theory can be a powerful tool to face the challenges of climate change, a topic on which we have interesting upcoming research.”
Padoan, S. A. and Stupfler, G. (2021). “Joint Inference on Extreme Expectiles for Multivariate Heavy-Tailed Distributions.” Bernoulli 28(2): 1021-1048 (May 2022).
https://doi.org/10.3150/21-BEJ1375
By Sirio Legramanti
Source: Bocconi Knowledge