The Math of Stem Cells
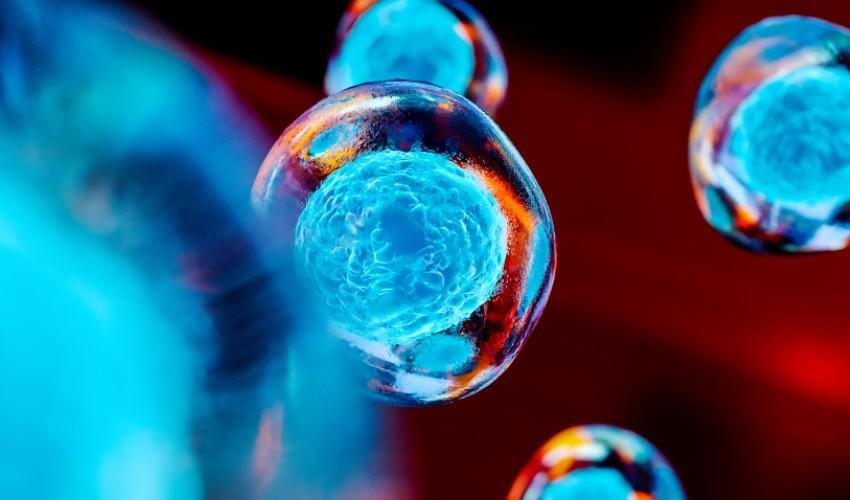
Stem cells are able to differentiate into various types of cells, such as muscle, epithelial or blood cells. New technologies like single-cell RNA sequencing allow to measure the state of each single cell at different stages of its evolution. However, analyzing a cell puts an end to its life, so that we cannot measure the same cell in two distinct moments of its differentiation process. This makes reconstructing the differentiation path of each stem cell very hard, a bit like guessing the whole movie from a few screenshots.
The solution of this intriguing biological problem requires refined mathematical tools, such as optimal transport theory. This branch of mathematics studies how to transport a distribution (of goods, mass, etc.) into another one, at the minimal cost.
(Find out more about optimal transport in this article about Bocconi Professor Giuseppe Savaré)
In the considered stem cell application, optimal transport is employed to connect distributions at consecutive timepoints in order to reconstruct whole paths from snapshots.
The problem is further complicated by the sparsity of the available data. In this case, sparsity comes from the fact that only few cells are measured at each timepoint. An existing algorithm by Geoffrey Schiebinger and colleagues can return some useful output when many cells are observed at each timepoint, but cannot handle the sparse scenario and hence is less helpful in practice.
This is where the mathematical abstraction developed by Hugo Lavenant, Assistant Professor at Bocconi Department of Decision Sciences, comes to rescue. In collaboration with Schiebinger himself and with Young-Heon Kim and Stephen Zhang, all from the University of British Columbia, Lavenant provided a modelling framework for cell differentiation paths through stochastic differential equations, thus justifying the use of optimal transport in the non-sparse setting and making inference possible even in the more challenging sparse scenario.
“This shows how powerful a mathematical abstraction can be”, explains Lavenant. “It took me some time to learn about this biological problem and give it a precise mathematical formulation, but it was a very good time investment. It is very satisfying when the math that you have developed allows to tackle another level of complexity in the application, and – on the other hand – applications push you to investigate new theoretical problems that may be useful in other settings”.
“For example, this stem cell application has stimulated us to work on unbalanced optimal transport, in which some mass can get lost or created between the starting and ending distribution. In this biological application, such a mathematical ‘complication’ is needed to model the fact that – along their differentiation process – cells may duplicate or die. This also poses mathematical questions which are very interesting in themselves, for which we have promising preliminary results that may contribute to optimal transport theory in general. It’s like an endless back-and-forth between applications and theory, enriching both sides.”
Find out more
H. Lavenant, S. Zhang, Y.-H. Kim and G. Schiebinger (2021) “Towards a Mathematical Theory of Trajectory Inference.” Preprint on arXiv: https://arxiv.org/abs/2102.09204
H. Lavenant (2021). “Unconditional Convergence for Discretizations of Dynamical Optimal Transport.” Mathematics of Computation, 90(328), 739-786. https://doi.org/10.1090/mcom/3567
H. Lavenant, S. Claici, E. Chien, & J. Solomon (2018). “Dynamical Optimal Transport on Discrete Surfaces.” ACM Transactions on Graphics, 37(6), 1-16. https://doi.org/10.1145/3272127.3275064
G. Schiebinger, et al. (2019). “Optimal-Transport Analysis of Single-Cell Gene Expression Identifies Developmental Trajectories in Reprogramming.” Cell, 176(4), 928-943. https://doi.org/10.1016/j.cell.2019.01.006
by Sirio Legramanti
Source: Bocconi Knowledge