Bocconi Doctoral Students Win ASA Award
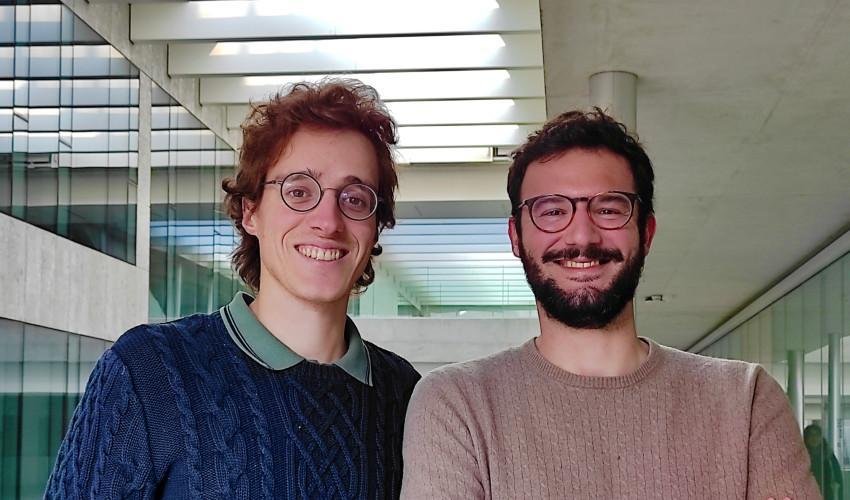
Filippo Ascolani (PhD candidate in Statistics) and Francesco Gaffi (PhD alumnus and currently postdoctoral fellow at the University of Notre Dame, United States) are among the winners for 2023 of the SBSS Student Paper Award, conferred by the American Statistical Association for innovative contributions in Bayesian statistics. Their work involves statistical models for data featuring a form of dependence known as partial exchangeability, which is appropriate when one wants to aggregate information from different sources or generated under differing experimental conditions. A classic example is the study of patients with a certain disease but treated in different hospitals, who may therefore present some level of heterogeneity.
Ascolani's paper, “Nonparametric Priors with Full Range Borrowing of Information”, co-written with Beatrice Franzolini (PhD Bocconi alumna and now a post-doc in Singapore), Antonio Lijoi and Igor Prünster (of Bocconi's Department of Decision Sciences), studies the implications of Bayesian statistical models in terms of relationships between groups of observations. In particular, a new measure of dependence, called hypertie, is introduced that captures both positive and negative associations between groups. This allows researchers to define a more flexible class of models that can incorporate a very broad spectrum of forms of dependence between sets of observations. As an example, different classes of financial instruments, such as stocks and bonds, may show opposite behaviors depending on market conditions.
In Gaffi's paper “Partially exchangeable stochastic block models for multilayer networks”, co-written with Daniele Durante (Assistant Professor in the Department of Decision Sciences), Antonio Lijoi and Igor Prünster, the same statistical assumption of partial exchangeability is used to build a model for relational data. In such a framework, the observational data consist of the connections between nodes in a network: typical examples are social networks, where nodes represent accounts, while connections may represent friendship, following, retweets, and so on. The model was created to deal specifically with multilayer networks in which nodes are naturally divided into different groups, such as criminal networks divided by geographic influence, or political networks divided by party affiliation. This new methodology makes it possible to infer a clustering of nodes that takes into account both this natural division and the connectivity of the nodes, as well as to predict the connections of new nodes that may join the network in the future.
The award will give Ascolani and Gaffi the opportunity to present their work at the 2023 Joint Statistical Meeting next August in Toronto, Canada.
Full article on Bocconi Knowledge