Marta Catalano and Sirio Legramanti Awarded for Their Papers
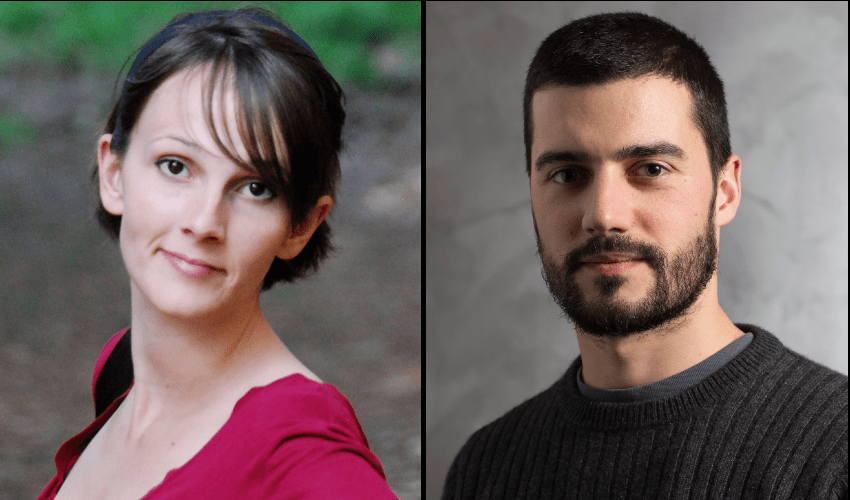
Marta Catalano and Sirio Legramanti, two Bocconi PhD candidates in Statistics, are among the ten winners of the SSBS 2021 Student Paper Competition, an award presented by the Section on Bayesian Statistical Science of the American Statistical Association (ASA).
Their papers have been selected for presentation at JSM 2021, the ASA Conference to be held in Seattle, 7-12 August, 2021, pandemic conditions permitting. The selected students will receive financial support for travel expenses. Furthermore, among the ten papers, the winner of the Laplace Award for the best student paper will be chosen.
“Congrats to Marta and Sirio for such an amazing achievement,” Antonio Lijoi, Bocconi PhD in Statistics Director, says. “It’s an important recognition of the breadth and novelty of their research, even more so if one takes into account that since 2015 only 15% of PhD candidates who have won the competition were from universities outside the US and Bocconi is the only university with two winners among the ten PhD students awarded for 2021.”
When modeling a structured experiment or a composite phenomenon, data from different but related sources are often available. For example, this happens when analyzing the clinical trials of a COVID-19 vaccine in different countries or the effects of a certain policy adopted by multiple regions. Several Bayesian models effectively leverage this valuable knowledge but need careful calibration. The amount of borrowing of information across different data sources ultimately amounts to a precise understanding of their dependence. In her paper “Measuring Dependence in the Wasserstein Distance for Bayesian Nonparametric Models” coauthored with Antonio Lijoi and Igor Pruenster, Marta Catalano singles out an ideal framework (based on the Wasserstein distance) for unraveling the dependence between complex datasets.
In his paper “Bayesian cumulative shrinkage for infinite factorizations”, coauthored with Daniele Durante and David B. Dunson (Duke), Sirio Legramanti proposes a novel prior distribution, called cumulative shrinkage process. Prior distributions are one of the key tools in Bayesian statistics and allow to formally encode what the analyst knows or expects before processing the incoming data. In particular, this new prior favors more parsimonious models, thus facilitating interpretation and speeding up computations, and has both theoretical and practical advantages over current competitors.
Source of the article: Bocconi Knowledge